PHOTO
We have heard much recently of the significant investment (and success) in building machine learning capabilities. Financial institutions are now striving to explore the full potential of artificial intelligence agents to replicate (and improve upon) human decision-making. With fraud detection, for example, the hope is to reduce the number of false claims and instances of money laundering. With trading, the hope is to reduce the number of underestimated risks and overestimated returns. With robo-advisors, the hope is to reduce research costs and portfolio misallocations.
Ideally, machine learning will produce superior outcomes at lower cost. However, to what extent will machine learning capabilities avoid repeating human mistakes? As we delegate more of our decisions to machines, can we look to eliminate more of our own cognitive biases? We need to first understand them much better.
Bias is natural but, out of nature, often unhelpful
The human brain is very powerful but has, of course, limitations. For example, it struggles to process fast-changing or large amounts of data. It therefore developed many decision simplification filters and as a result, evolved to rely on complex forms of bias. These biases help it assess and rationalize risks to determine which it can accept and which should be avoided. Human societies now are more complex, and individuals face different threats. They also face more and different decisions, but our cognitive abilities need much more time to evolve similarly.
When faced with too much information with too limited experience, we too often simply guess. This is true even for experts, and it’s interesting when applied to financial professionals. The trouble for the rest of us comes when experts are blind to their blindness. Their cognitive biases can create errors which can then compound and potentially contribute toward systemic risk if they are used in developing, say, economic policies and financial supervision strategies or informing regulatory enforcement.
Our decisions are based on validated facts as well as unsupported assumptions. The result is we don’t realize this is happening or the implications.
Unconscious bias
This is often called the mother of all biases because it is often a component of many other biases. We may – often without realizing it – accept or dismiss an investment proposal based on preconceptions of the people proposing them. To save time and effort, we pigeonhole proposers based on criteria such as age, gender and even perceived attractiveness. We also subconsciously assign presumed traits as to ability and even honesty.
We may be less rigorous with a proposal from a proposer whom we identify closely with, perhaps imagining a bond because we attended the same school or university. Alternatively, we may be overly rigorous with a proposer who didn’t attend university at all. While unintended, it can nonetheless prevent critical self-challenge and disciplined appraisal.
We both under- and overestimate some people, opportunities and risks based on entirely irrelevant factors. The result is that we unknowingly mis-price risk.
Confirmation bias
The key to its perniciousness is that we may seek out evidence that agrees with us and are much harder on evidence that doesn’t support what we wish to believe. When developing an investing thesis, we may overweight helpful anecdotal data and underweight unhelpful actual data. Similarly, we may seek out only those that we agree very well with which can lead to group think, prevent constructive challenge and can create fatal blind spots.
We overestimate our objectivity, as well as our efforts to be rigorous. The result is a false sense of security.
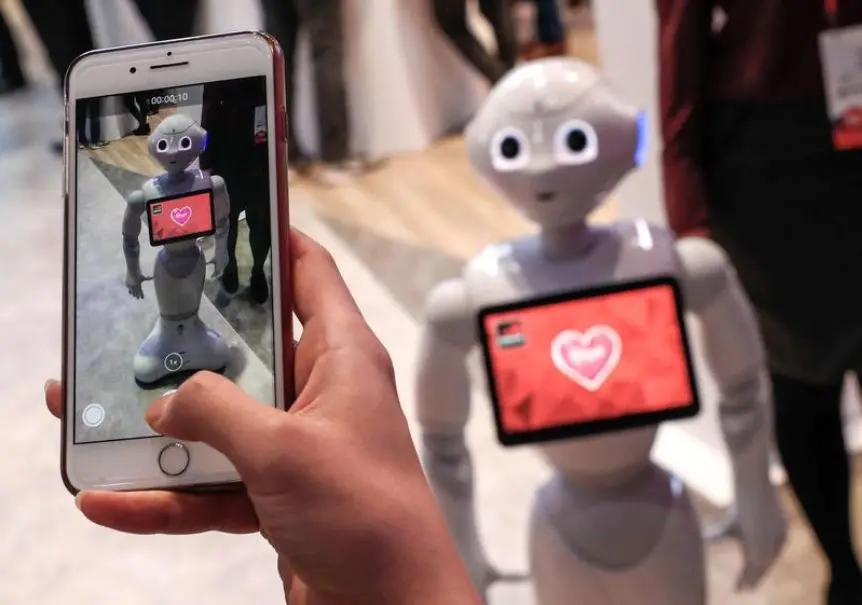
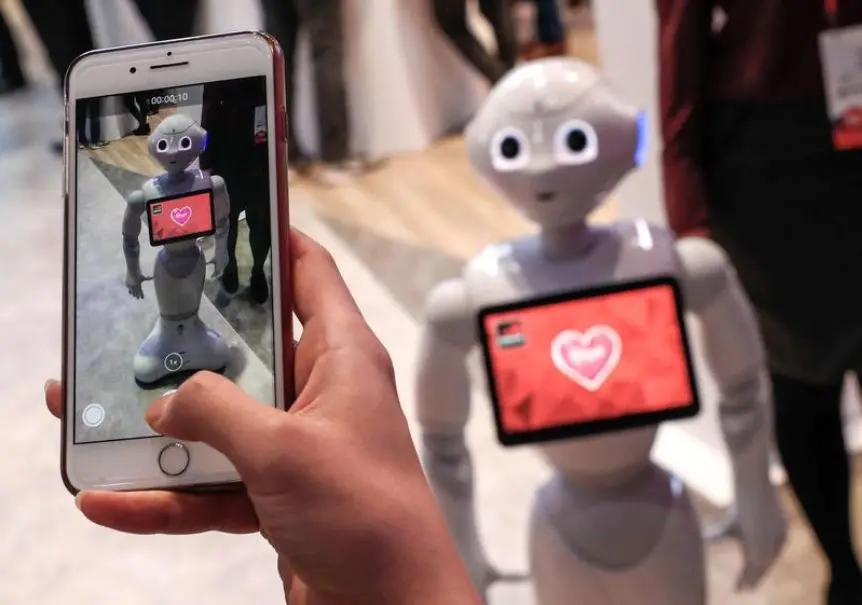